Bridging the gap between finance theory and practice is at the heart of a collaboration between MSCI ESG Research and the MIT Sloan Finance Lab, or Fin-Lab, a graduate-level course providing immersive learning for the school’s masters students.
The collaboration, which is supervised by Xinxin Wang, a fellow at the MSCI Sustainability Institute and a leader in ESG and climate research for the Americas, has produced a decade of research into questions ranging from the challenges of reducing the emissions of investments while continuing to invest in the energy sector to the relationship between sustainability strategies and risk-adjusted returns.
The collaboration has entered its second decade, with the latest cohort of students examining whether data from MSCI’s Climate Target and Commitments dataset, which evaluates a company’s climate commitments based on their comprehensiveness, ambition and feasibility, can serve as a meaningful tool for investors who are analyzing corporate bonds.
You can read more about the collaboration here.
Below is an overview of five Fin-Lab research projects supervised by MSCI since 2019.
Highlights from the last half-decade
Below is an overview of five Fin-Lab research projects supervised by MSCI since 2019.
Do low-carbon strategies pay off? (2024)
Researchers: Pincha Rutchatawuttipong, Jinghan Chen, Alexander Genoe, and Stella Kotzabasakis
MSCI supervisor: Xinxin Wang
The study explores the link between low-carbon strategies and financial performance in the energy and utilities sectors. Using regression analysis and performance difference methods, the research identifies signs of a positive correlation, highlighting investor pressure as a key driver. The findings underscore the challenges of data quality and the long-term nature of carbon transitions.
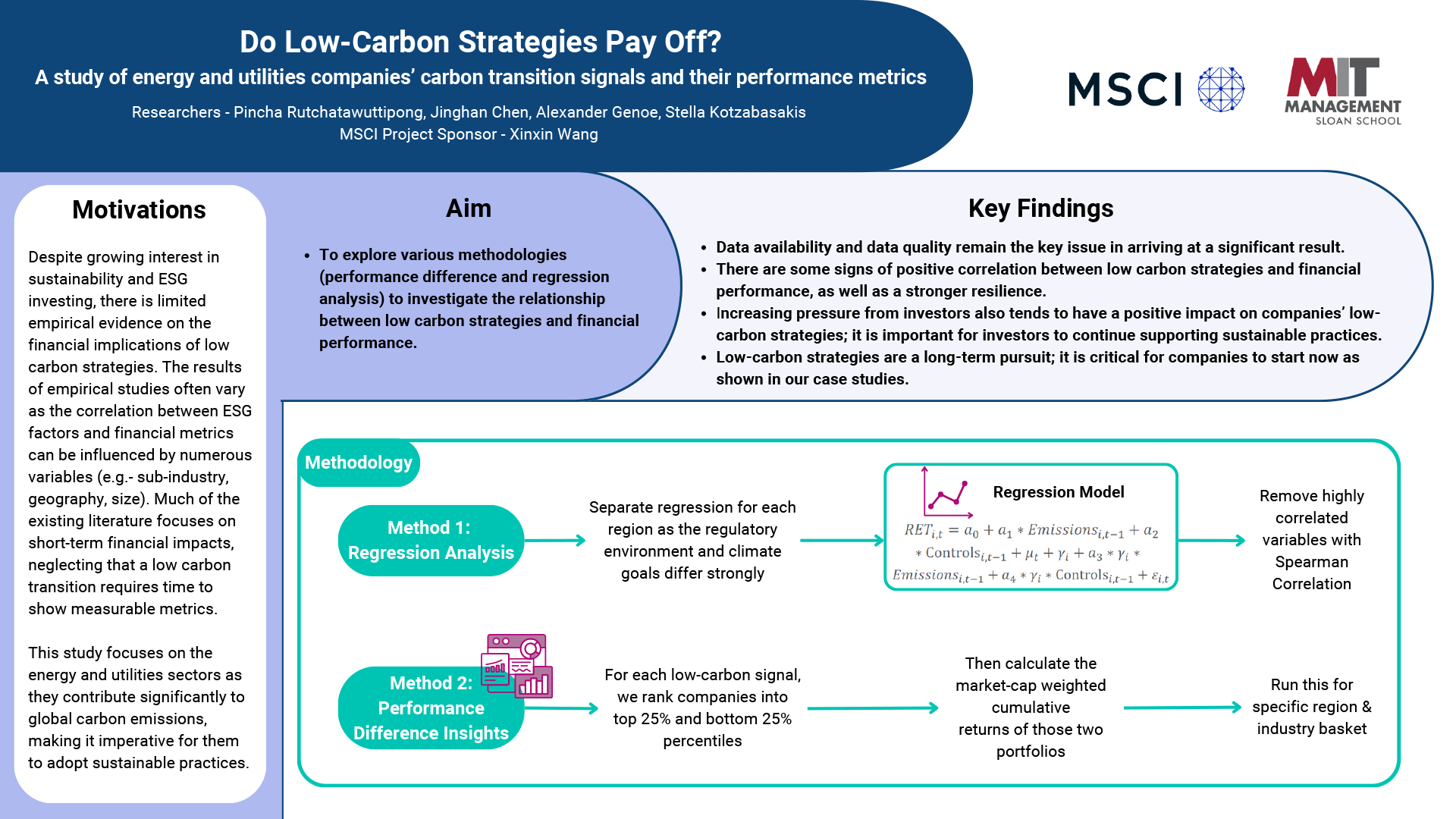
Low-carbon portfolio strategies (2023)
Researchers: Wenqing Zhang, Xinyan Xie, Aya Solombela, Xinyue Jiang
MSCI supervisor: Xinxin Wang
The study evaluates strategies for constructing low-carbon portfolios while maintaining material exposure to the energy sector. Researchers compare two models: a standard model designed to maximize expected return and a risk target model optimizing active return. Key findings reveal that maintaining energy assets can achieve decarbonization but may trade-off risk-adjusted returns. Challenges include data reliability and emissions volatility driven by underlying carbon data.
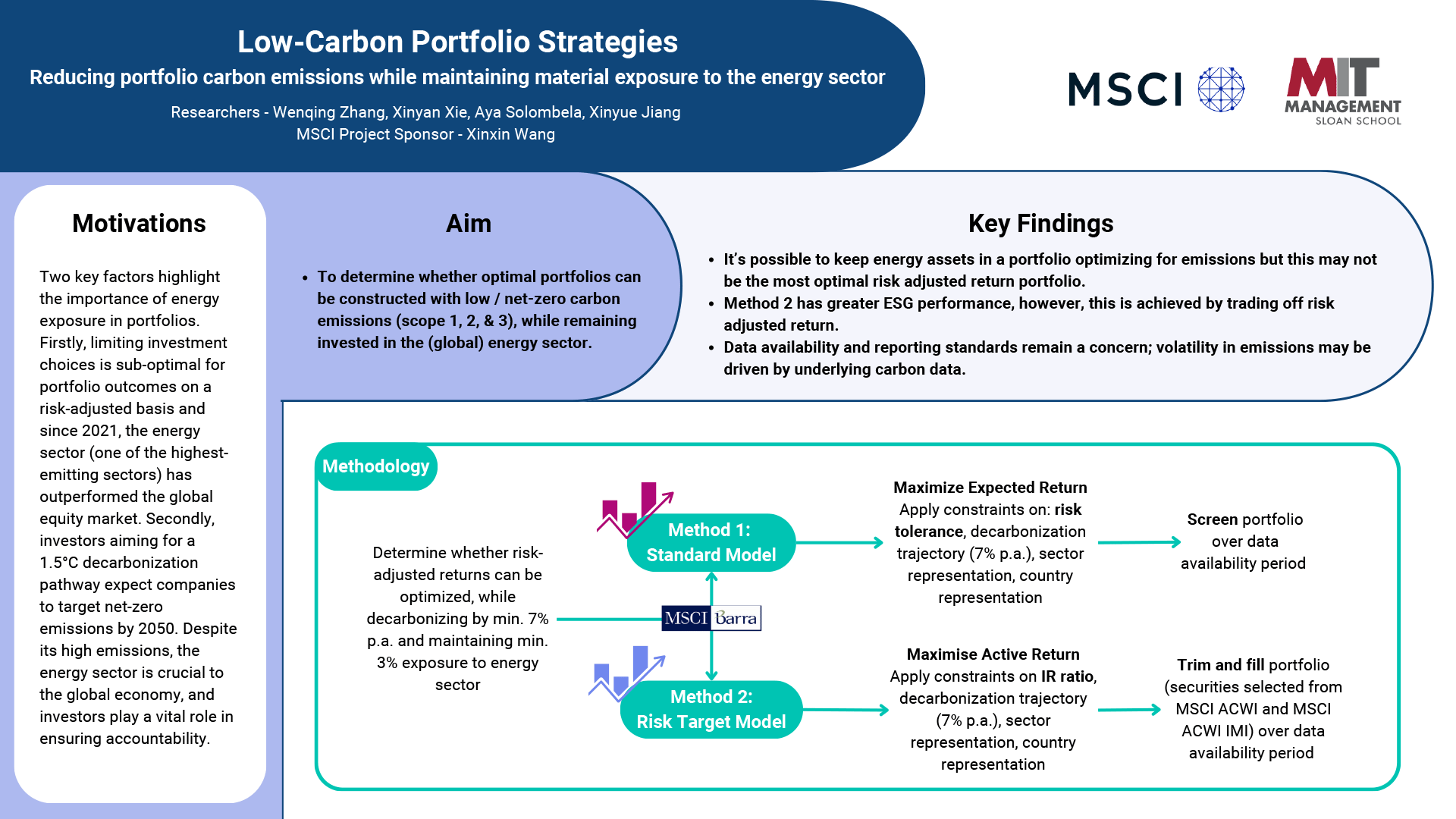
Risk-return trade-off with ESG constraints (2022)
Researchers: Rachel Qian, Liehan Jiang and Tianyi Zhang
MSCI supervisors: Xinxin Wang and Luis Vivas
The study analyzes the impact of ESG constraints on investment portfolio performance, exploring the balance between sustainability and profitability. It finds that well-imposed ESG constraints won’t necessarily reduce portfolio returns if imposed reasonably and ESG factors can be regarded as a fundamental factor that derives performance from superior earnings growth.
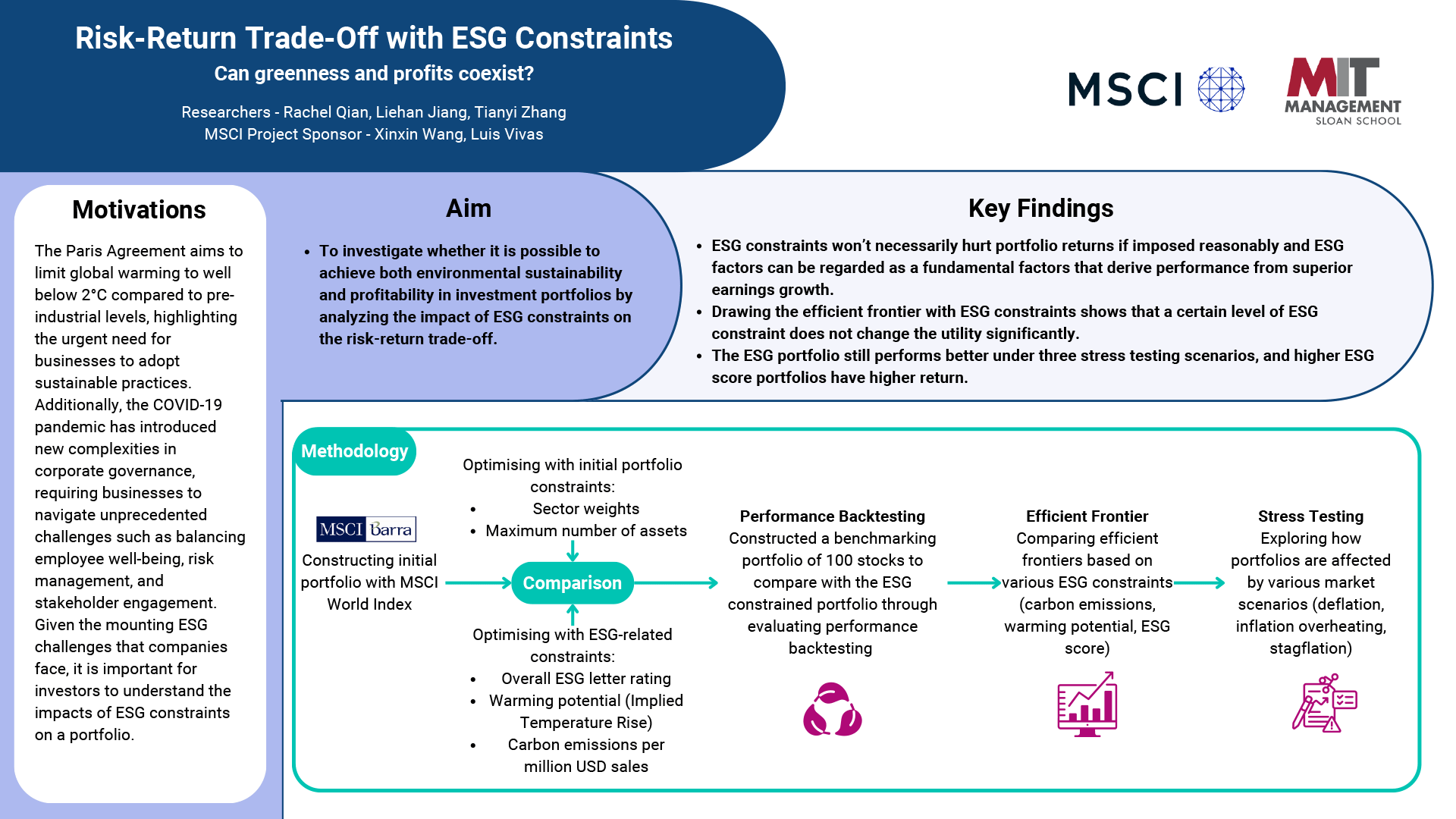
How markets view carbon commitments of companies (2021)
Researchers: Dongqi Guo, Sergio Miguel Moya Jimenez, Alina Shestiaeva
MSCI supervisors: Xinxin Wang and Oliver Marchand
The study analyzes the incidence and importance of carbon target emissions announcements on long-term stock price returns in the U.S.
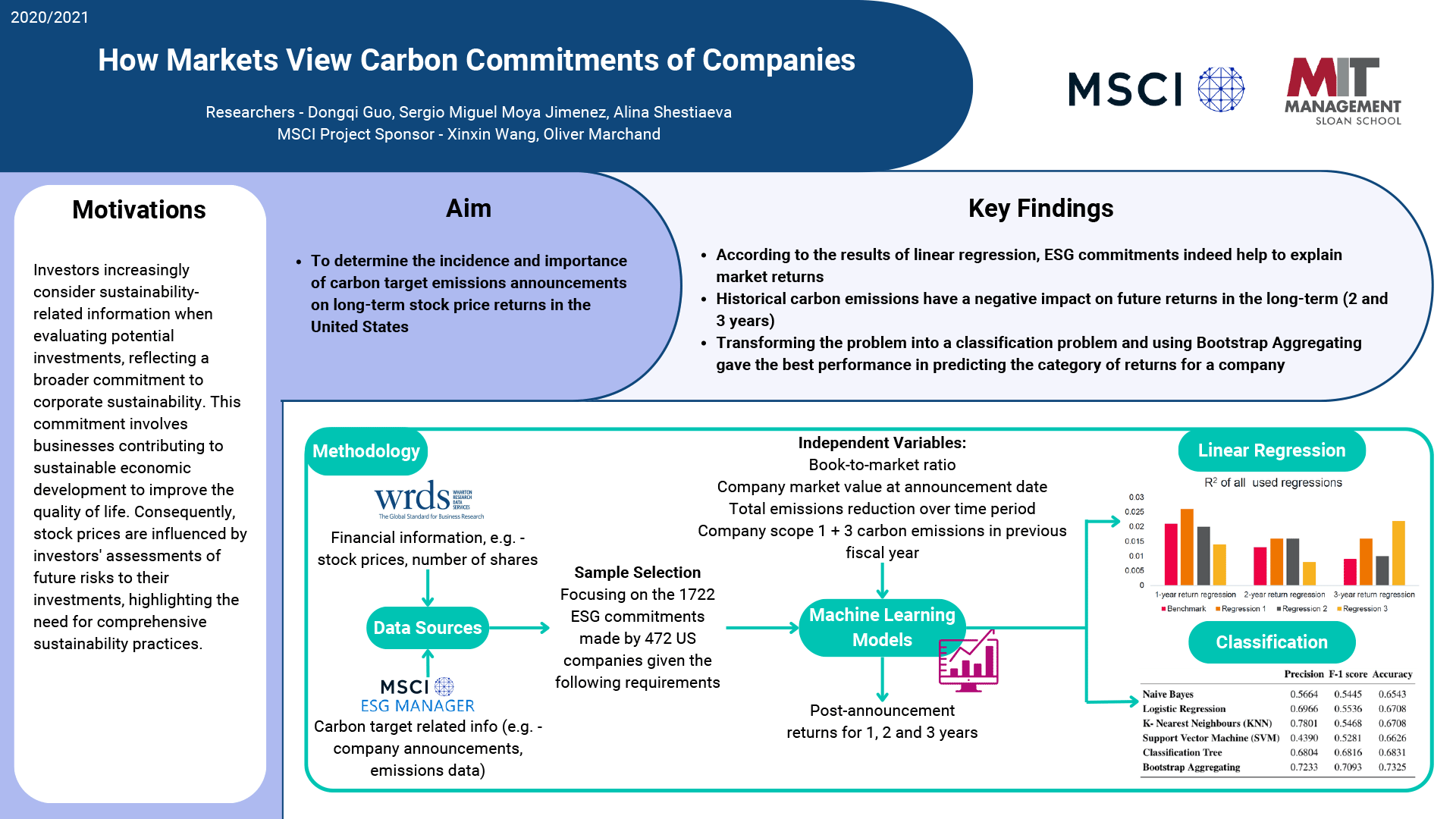
Identifying market-moving topics (2020)
Researchers: Jiada Chen, Michael Wehbe, Xiaopeng Wu
MSCI supervisor: Xinxin Wang
The study investigates the modeling technique Latent Dirichlet allocation (LDA) for identifying market-moving topics in 10-K reports. By analyzing topic evolution and linking them to security returns, the study shows how dominant topics influence market outcomes. The study emphasizes LDA’s value in managing large bodies of text, enabling systematic insights into business risks, market sentiment and alpha generation.
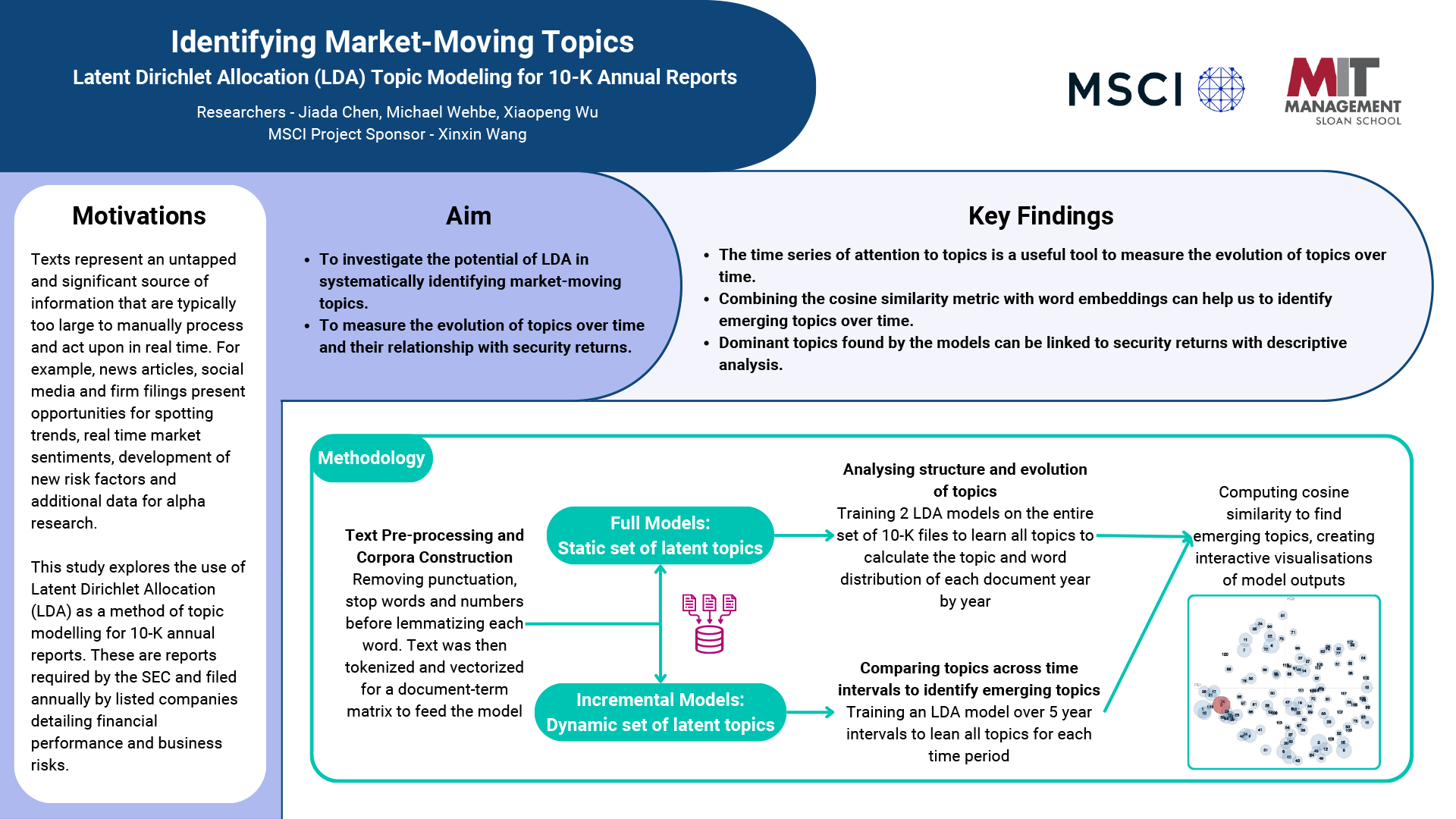
Portfolio optimization tools for fundamental funds (2019)
Researchers: Bolong Tan, Vanessa Guo, Yi Lu, Youwei Li
MSCI supervisors: Xinxin Wang, Dan Sinnreich, Ramarathnam Radhakrishnan
The study explores integrating quantitative tools with fundamental fund management following the 2008 financial crisis. Using optimization models and interviews, it finds that discretionary decisions outweigh quantitative inputs for fundamental fund managers throughout the investment process. The study develops an optimization model that improved risk-adjusted returns of funds with various coverages and finds that optimized portfolios reduce downside risk and outperform during downturns. The findings highlight the potential of combining traditional investment approaches with quantitative techniques to adapt to changing capital flows.
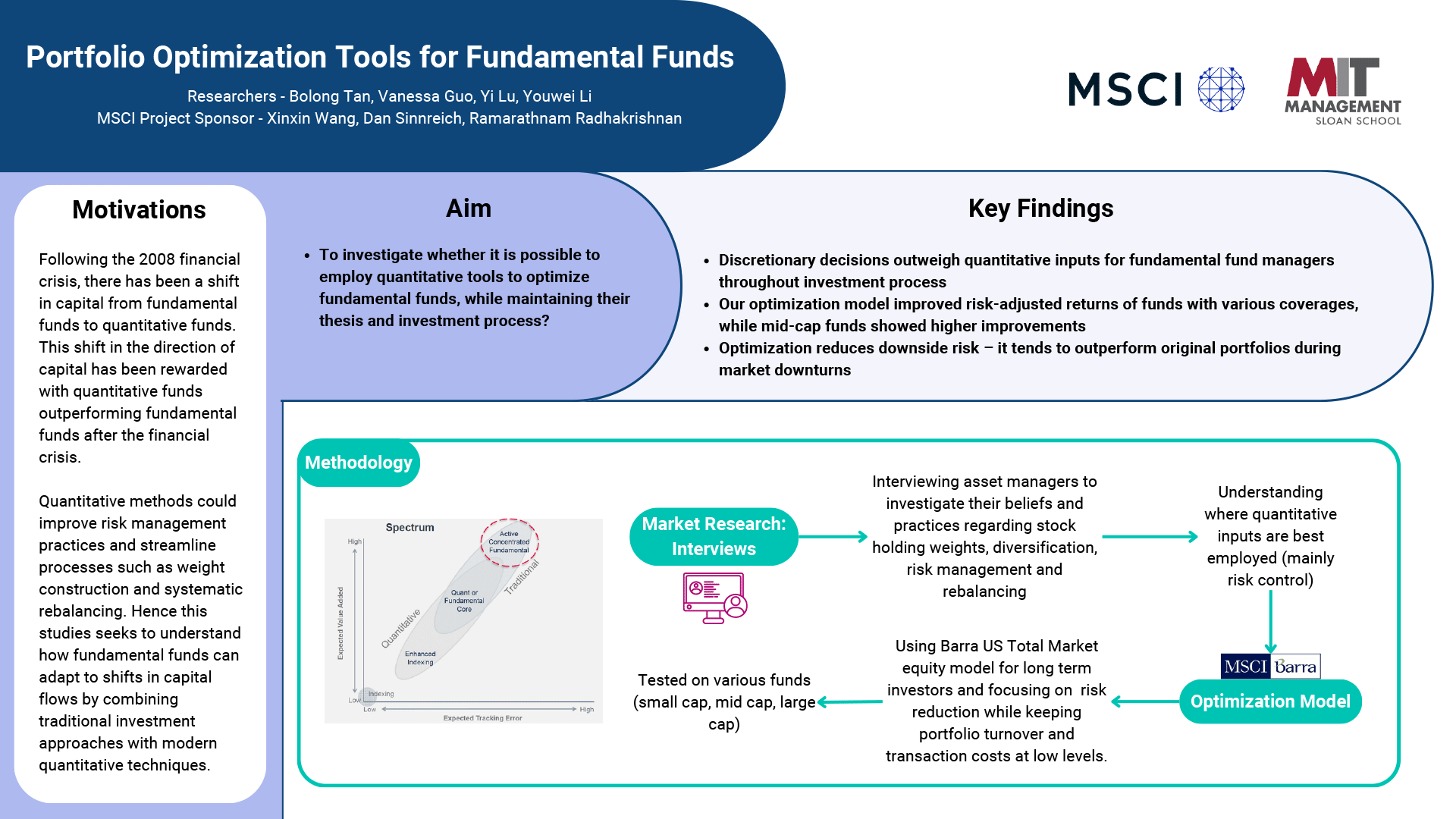